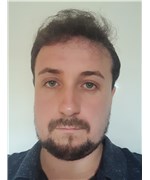
Machine learning-based assessment of novel biomarkers for aggressive prostate cancer
Project Abstract
Prostate cancer is an enormous health challenge, as it is the second-most commonly diagnosed cancer in men. At present, there is no diagnostic test that is both highly accurate and non-invasive being used in routine clinical practice. Extracellular vesicles (EVs) are a rich source of information about their cell of origin and are detectable in bodily fluids, such as urine and blood serum. Therefore, the analysis of the mRNA from the EVs is a means of carrying out a non-invasive, liquid biopsy. This research aims to create a robust machine learning model that is able to accurately predict if a person has prostate cancer and whether or not it is aggressive. It will utilise EV mRNA signatures and other non-invasively collected markers (such as prostate specific antigen). For the analysis of this genetic dataset, machine learning models are going to be used. Methods identified in the literature include Boruta feature selection, random forest classifiers and artificial neural networks. Due to its popularity in both research and industry, the sci-kit learn library will be the machine learning library of choice. If this project is successful, the resultant prostate cancer classifier will generalise to accurately predict and grade prostate cancer across datasets collected from different studies and under different conditions. Furthermore, to provide confidence to geneticists, the machine learning model should be explainable. The mRNAs determined as of the most importance to the classifier will be verified against genetic databases and other in-vitro studies.
Keywords: Prostate cancer, Machine Learning, Explainable AI
Conference Details
Session: Presentation Stream 18 at Presentation Slot 5
Location: GH014 at Wednesday 8th 09:00 – 12:30
Markers: Benjamin Mora, Jen Pearson
Course: MSc Computer Science 2yr PT, Masters PG
Future Plans: I’m undecided