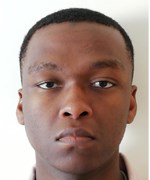
Machine Learning from Human Electroencephalogram Data
Project Abstract
Machine learning is the process of automatically generating and improving programs through algorithms. The programs generated seek to address problems on a case-by-case basis. Due to the diversity of real-world problems, machine learning does not provide a one size fits all approach. Different algorithms shine in different settings, and the desire to understand best practices in these settings is ever increasing. Traditionally, data accumulated has been processed manually by human operation. As technology has improved so has humanity?��s ability to process information, by organising it, visualising it and manually gaining insights from it. But these means are reaching their limits. Machine learning offers a place where a greater number of insights can be gained under the greater strain of computational issues. Therefore, this project addresses a multiple independent variable scenario to deduce which machine learning algorithm, in combination with selected pre-processing methods, will provide the greatest accuracy for classification of states of the mind. The study took sets of data obtained through EEG and established the best algorithms for predicting what state of mind an individual may be in. Through process of elimination, it was discovered that K nearest neighbours, Support Vector machine, Random Forest and Gradient Boosting were the algorithms that performed the best under multiple classification scenarios. Their robustness invited further study into creating a single pipeline with a single machine learning classifier that would accomplish the task to the highest degree.
Keywords: Machine Learning, Multivariate Problems, Human EEG Data
Conference Details
Session: Poster Session A at Poster Stand 53
Location: Sir Stanley Clarke Auditorium at Tuesday 7th 13:30 – 17:00
Markers: Jiaxiang Zhang, Arno Pauly
Course: BSc Computer Science, 3rd Year
Future Plans: I’m looking for work