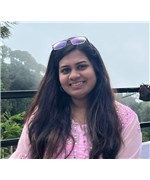
Comparative Analysis of ML algorithms for Anomaly Detection in the In-Vehicle Networks with Matthew?��s Correlation Coefficient
Project Abstract
As cyber-physical systems become more integrated, the automobile sector needs strong cybersecurity solutions to protect car networks from cyberattacks. In response, this project advises testing machine learning (ML) algorithms for in-vehicle network anomaly detection. The Matthews Correlation Coefficient (MCC) will be used to evaluate machine learning methods such as isolation forest, logistic regression, mini-batch K-means clustering, BIRCH, and multi-layer perceptron for real-time automotive cybersecurity threat detection. This research project will use MCC, known for its reliability in imbalanced datasets from cybersecurity scenarios, to compare ML algorithms. The project also shows how machine learning-based anomaly detection can improve automotive system safety and cyberattack resistance. This will assist in developing automotive cybersecurity-adaptive intrusion detection systems. This project seeks to provide practical insights into real-time fault detection and intrusion detection system enhancement in the automobile industry using machine learning methods.
Keywords: Anomaly Detection, ML Algorithm Evaluation, In-Vehicle Networks
Conference Details
Session: Presentation Stream 23 at Presentation Slot 5
Location: College 127 at Wednesday 8th 09:00 – 12:30
Markers: Eike Neumann, Julian Hough
Course: MSc Cyber Security, Masters PG
Future Plans: I’m looking for work