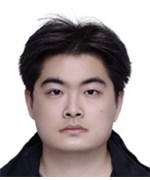
Enhancing the Usability of EEG-Based Biometric Recognition Systems
Project Abstract
Electroencephalographic (EEG) signals hold immense potential for secure biometric identification due to their inherent complexity and resistance to forgery. This project explores the usability of EEG signals within biometric recognition, addressing significant challenges such as user comfort, signal acquisition, and data processing. Our research, grounded in the usability limitations of current EEG technology, aims to develop a user-friendly identification system that ensures security and convenience.The proposition of this study lies in its novel approach to assess EEG signal viability, emphasizing the reduction of electrode complexity and improvement of signal processing methods. By innovating lightweight EEG headsets and implementing advanced machine learning techniques, this research occupies a unique position in the intersection of biometric security and neurotechnology.Employing a mixed-methods approach, we synthesized insights from commercial EEG devices, academic literature, and experimental trials. We leveraged a minimal electrode setup and harnessed cutting-edge algorithms for feature extraction and signal classification. This interdisciplinary method provides a comprehensive understanding of EEG’s biometric applications, including the stability of EEG patterns over time?��a phenomenon known as template aging.Our preliminary findings indicate that EEG signals, when processed with machine learning algorithms, can achieve high authentication accuracy. Notably, user comfort directly correlates with the quality of EEG data, highlighting the importance of headset design and electrode configuration in the practical deployment of EEG-based systems.In conclusion, this research marks a significant step toward the practical use of EEG for biometric identification. Although findings are yet to be conclusive, they suggest a promising future for EEG biometrics, contingent on overcoming challenges related to usability, signal stability, and data security. Further work will focus on long-term usability studies, ensuring that EEG-based biometric systems can become an integral part of secure identification protocols.
Keywords: Big Data, Cyber Security, Machine Learning
Conference Details
Session: Presentation Stream 24 at Presentation Slot 5
Location: College 017 at Wednesday 8th 09:00 – 12:30
Markers: Fabio Caraffini, Nader Al Khatib (GTA)
Course: MSc Advanced Computer Science, Masters PG
Future Plans: I’m looking for work