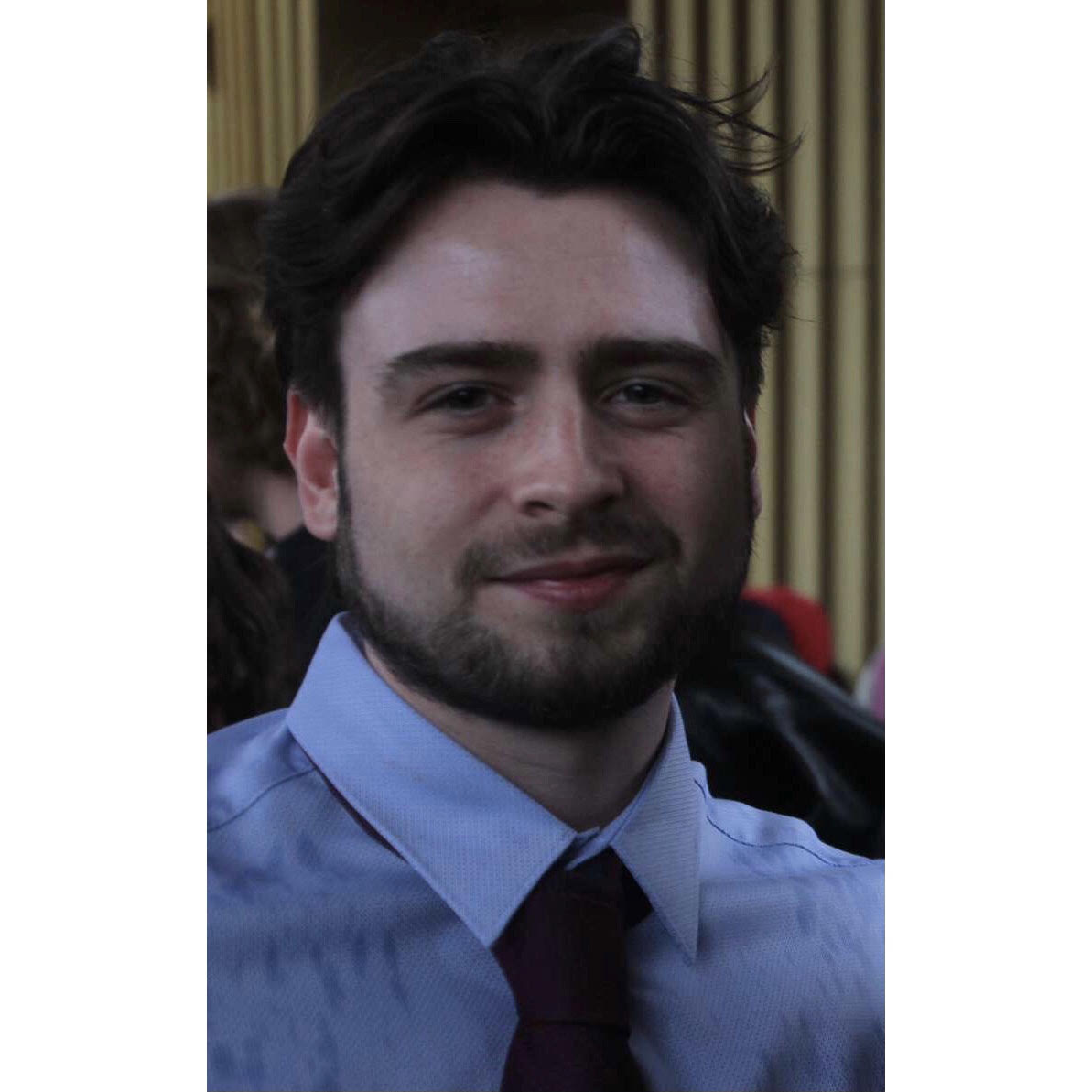
Two-Stage Brainwave-Based Person Recognition System
Project Abstract
Traditional security methods like passwords and fingerprint biometrics have proven vulnerable in today?��s ever-evolving digital landscape, emphasizing the need for more secure authentication solutions. This project proposes a two-stage person recognition system using electroencephalography (EEG) signals which leverages the chaotic and unique nature of brainwave signals as a unique biometric form of recognition. Our methodology centres on evaluating various machine learning techniques such as Support Vector Machines and Random Forest to optimally extract and classify EEG features. We aim to utilize a two-stage verification process to further enhance our security and to limit the rates of false acceptance and rejections with a focus on spoofing attacks, which has been shown to be possible on previous EEG-based systems. We evaluate efficient placement for EEG channels and effective feature extraction to optimize the system?��s accuracy and efficiency across a large data set. Our research expects to demonstrate that efficient channel placement and feature extraction techniques can significantly improve our system’s ability to authenticate users and when combined with two-stage authentication can prove resilient against spoofing attacks across a large range of users, setting a new standard in biometric security. Upon completion, we expect to contribute a significant new insight into the field of security by demonstrating how an EEG-based recognition system, enhanced by advanced machine learning techniques and optimal channel placement, can provide a promising solution to the limitations of current authentication methods
Keywords: Machine Learning, Big Data, Security
Conference Details
Session: Presentation Stream 23 at Presentation Slot 4
Location: College 127 at Wednesday 8th 09:00 – 12:30
Markers: Eike Neumann, Julian Hough
Course: MSc Advanced Computer Science, Masters PG
Future Plans: I’m looking for work