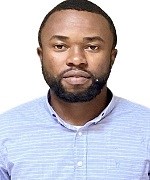
Energy Consumption Prediction Using XGBoost and Quantile Regression: An Integrated Approach for Feature Selection and Interval Forecasting
Project Abstract
Over the years, exponential population growth, economies, and rapid industrialisation have contributed to the alarming increase in global energy demand. The U.S. Energy Information Administration reported that the building sector consumes 23% of worldwide energy, ranking third after industry (37%), and transportation (28%). Thus, accurate prediction of building energy consumption is crucial for effective energy management and planning. However, despite the advancement in Machine Learning (ML) for energy prediction, traditional models often fall short in performing interval prediction and feature extraction procedures, especially amidst high data uncertainty. Thus, this study proposes a unique data-driven XGBoost-Quantile Regression (XGBoost-QR) hybrid approach, leveraging the strengths of both methods to simultaneously implement embedded feature selection and parameter learning, and apply it to point prediction and interval prediction of building energy consumption.The proposed XGBoost-QR hybrid model capitalizes on the inherent feature selection capabilities of XGBoost and the interval prediction strength of QR to select the most representative subset of features from the training set. The proposed model parameter optimization is achieved through Bayesian optimization while cross-validation will be implemented in the model training process to ensure that it generalizes well to unseen data.The robustness of the proposed model will be tested under various conditions, and its predictive accuracy and efficiency performance will be benchmarked against other standard and hybrid models using metrics such as Root Mean Square Error (RMSE), Mean Absolute Percentage Error (MAPE), Coverage Probability Percentage (CPP), and Mean Width Percentage (MWP). This study’s contribution lies in its potential to outperform existing predictive models, offering a significant advancement in energy management and planning.
Keywords: Building Energy Consumption, XGBoost-Quantile Regression, Machine Learning
Conference Details
Session: Presentation Stream 12 at Presentation Slot 1
Location: College 017 at Tuesday 7th 13:30 – 17:00
Markers: Scott Yang Yang, Ehinafa Akinola (GTA)
Course: MSc Computer Science, Masters PG
Future Plans: I’m looking for work