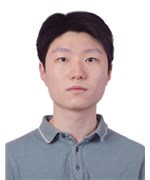
Adversarial Attacks in image classification
Project Abstract
As electronics grow increasingly intelligent and connected to the internet, fresh security concerns emerge. In deep learning, adversarial attacks is a one the means of jamming. In academic research, it has been identified that deep learning systems are susceptible to adversarial attacks, wherein hostile entities introduce subtle perturbations to images. These perturbations, often imperceptible to the human eye, can precipitate a marked degradation in the predictive accuracy of such systems. For example, Driverless technology is based on deep learning, and if the driverless driver is interfered with by an adversarial attack, it will not be able to identify vehicles or pedestrians. As a result, it is impossible to brake or avoid in time, resulting in unavoidable losses caused by car accidents. So it is not difficult to see that deep neural networks(DNN) is easy to be attack. The main objective of this paper is to investigate the classification of traffic signs on roads by means of convolutional neural networks. Then FGSM attack is performed to reduce its classification accuracy. Of course, based on the previous literature of this research, this paper also discusses the main challenges and future perspectives and possible solutions in this direction. It has been proven that after Fast Gradient Sign Attack, the accuracy rate of traffic sign classification is greatly reduced. This means that although deep learning can bring a lot of convenience to human beings, it still needs to improve its anti-interference ability to be competent for more work in the future.
Keywords: Machine learning, Convolutional neural network, Fast Gradient Sign Attack
Conference Details
Session: Poster Session B at Poster Stand 129
Location: Sir Stanley Clarke Auditorium at Wednesday 8th 09:00 – 12:30
Markers: Lu Zhang, Jay Morgan
Course: BSc Computer Science, 3rd Year
Future Plans: I’m continuing studies