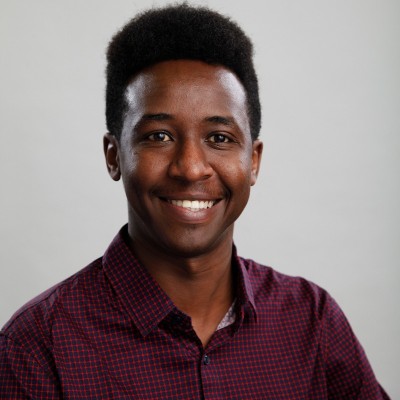
Leveraging Generative Adversarial Networks for Satellite Crop Image Analysis
Project Abstract
With the growing global population and the effects of climate change, timely and accurate global-scale maps of crop type distribution are crucial for monitoring food security, assessing environmental changes, and planning sustainable land management practices. This research addresses the challenges faced by deep learning models in land monitoring using satellite data due to imbalanced real-world data distributions of land cover and crop types. However, the imbalanced distribution of real-world data poses significant challenges to the scalability and transferability of deep learning models in this context. Although advancements in spatial and temporal accuracy have improved, certain regions still exhibit a tendency to grow specific types of crops, exacerbating the class imbalance problem. The goal of this paper is to address this issue by using Generative Adversarial Networks (GANs) to produce synthetic images for under-represented crop types. Furthermore, we will investigate the possibility of utilizing the discriminator part of GAN for crop type classification. Through this, we aim to enhance the precision and reliability of crop type analysis models, enabling better decision-making in agricultural management practices.
Keywords: Generative Models, Deep Neural Networks, Artificial Intelligence
Conference Details
Session: Presentation Stream 7 at Presentation Slot 2
Location: GH018 at Tuesday 7th 13:30 – 17:00
Markers: Sofya Lyakhova, Troy Astarte
Course: MSc Advanced Computer Science, Masters PG
Future Plans: I’m looking for work