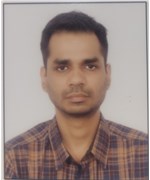
Automation the In Vitro Micronucleus Assay
Project Abstract
Motivation:- In vitro micronucleus testing is an important tool in toxicology that is used to evaluate the genotoxic potential of various medicines and materials. As a chromosomal damage indicator, micronuclei?��small additional nuclei formed during cell division, are major indicators for identifying whether a chemical has the potential for occurrence mutations or cancer. The development of micronuclei is a sign of genetic instability in nuclei, a very common feature to the development of cancer. Examining histopathology slides under a microscope is the traditional method of identifying and categorizing different malignancies. Skilled pathologists are usually responsible for this activity. It has been shown that relying only on visual inspection of well-established histological patterns is not always accurate or consistent, especially when it comes to detecting specific tumor subtypes and stages. This method can be time-consuming. It is hard to develop picture categorization algorithms that function consistently without human intervention or modification. The lighting, focus, and staining technique of the photographs we obtain will never all be the same, even if we standardize the processes.Aim:- The aim of this research is to develop and validate an automated micronuclei categorization system based on deep learning algorithms. Specifically, by combining the ability of advanced machine learning algorithms with image flow cytometry, we want to increase the result, effectiveness, and interlaboratory reproducibility of the cytokinesis-block micronucleus (CBMN) assay. Methods:- Automated techniques offer a feasible substitute by employing deep learning algorithms to enhance efficiency and dependability. In this work, we evaluate the performance of various deep learning algorithms, including DeepFlow Neural Network, YOLO, YOLO Lite, GoogleNet, and VGG16, for inter-laboratory scoring automation of the CBMN assay. Through careful research, we hope to ascertain the most effective method for a reliable and accurate assessment of genetic toxicity.Future Contribution:- Making tools for micronuclei classification with machine learning more accessible will improve the usefulness of these assays in industry, research, and clinical settings. Our research enhances automated cytogenetic analysis by tackling the challenges of manual scoring and harnessing modern computational methods. This ultimately results in more precise and effective assessments of chromosomal damage and genetic toxicity.
Keywords: Detection and Classification of Micro- Nuclei, Automation, deep learning, Cancer detection, Imaging Flow Cytometry (IFC)
Conference Details
Session: Presentation Stream 7 at Presentation Slot 5
Location: GH018 at Tuesday 7th 13:30 – 17:00
Markers: Sofya Lyakhova, Troy Astarte
Course: MSc Data Science, Masters PG
Future Plans: I’m looking for an industry placement