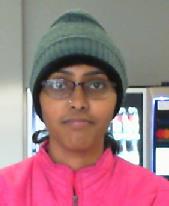
Medical Image Classification with Convolutional Neural Network
Project Abstract
This research explores the application of Convolutional Neural Networks (CNNs) in the classification of medical images, specifically lung image patches with interstitial lung disease (ILD). Given the increasing complexity of medical imaging applications and the need for accurate and efficient classification methods, this project work addresses the challenge of distinguishing between various ILD categories. The main aim of this study is to develop a customized CNN architecture tailored for image-like multi-class classification tasks in medical imaging, aiming to achieve higher accuracy and efficiency compared to traditional methods. Project approach involves the utilization of CNNs with a single convolutional layer, inspired by DCT-like kernel matrices, to effectively extract discriminative features from lung image patches. The experimental results demonstrate that project proposed CNN model outperforms existing approaches, achieving superior classification performance. Notably, project findings contribute to advancing the field of medical image classification by providing insights into the efficacy of CNNs for ILD pattern recognition, highlighting the potential for improved diagnosis and treatment planning in pulmonary diseases.
Keywords: Deep Learning, Pytorch, Convolutional Neural Networks
Conference Details
Session: Presentation Stream 5 at Presentation Slot 10
Location: GH011 at Tuesday 7th 13:30 – 17:00
Markers: Nader Al Khatib (GTA), Tom Owen
Course: MSc Computer Science, Masters PG
Future Plans: I’m looking for an industry placement